DeepSeek: The AI Disruptor That Shook the Tech World
- Parth Goel
- Feb 13
- 4 min read
On the day of Donald Trump’s presidential swearing-in ceremony, a quiet yet seismic shift occurred in the tech world. DeepSeek, a Chinese AI app, launched its groundbreaking R1 model, sending shockwaves through global markets and challenging industry giants like OpenAI. With its unparalleled efficiency, innovative strategies, and cost-effective approach, DeepSeek has redefined what’s possible in artificial intelligence.
While DeepSeek’s reduced computational costs lower AI’s carbon footprint, its success could lead to increased AI consumption, potentially offsetting these environmental benefits. This duality raises important questions about the future of sustainable AI development.
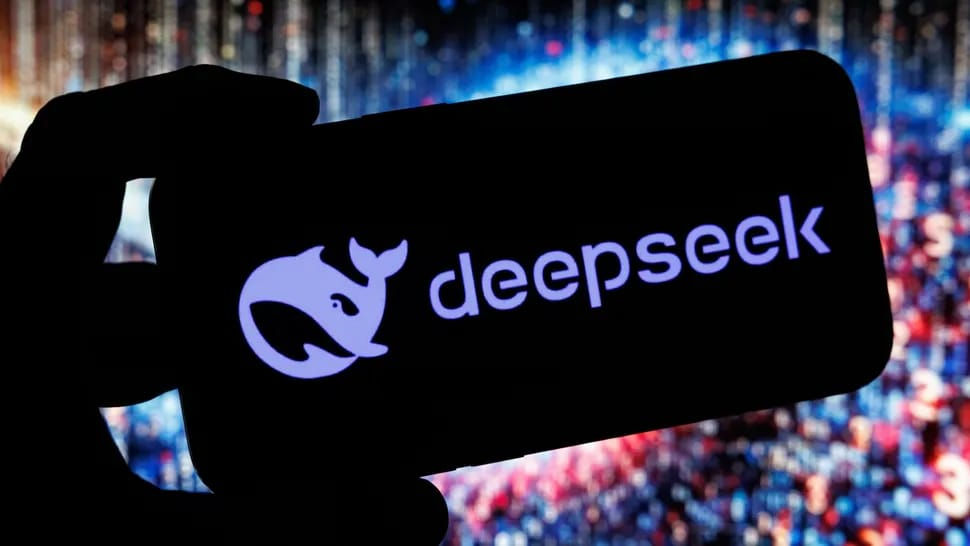
Key Features of DeepSeek
DeepSeek’s rise to prominence is built on several groundbreaking features that set it apart from its competitors:
28x Cheaper:
DeepSeek’s R1 model rivals OpenAI’s GPT-4 in reasoning capabilities but is 28 times cheaper to train and operate. This cost efficiency has disrupted the industry, forcing competitors to rethink resource-heavy approaches.
Lean Team:
With just 200 employees, DeepSeek has achieved what many thought was impossible for a small team. In contrast, OpenAI employs over 4,000 people. This lean structure highlights DeepSeek’s ability to innovate efficiently without the need for massive manpower.
Open-Source Model:
DeepSeek has embraced open-source development, making its models accessible to the public. This democratizes AI, fosters collaboration, and challenges the proprietary models of competitors like OpenAI and Google’s Gemini.
Idle GPU Usage:
DeepSeek optimized its training process by leveraging idle GPUs, reducing computational waste and further cutting costs. This innovative approach maximizes resource efficiency, a key factor in its low development budget.
Trained on 2,000 Nvidia H800 GPUs:
The R1 model was trained on 2,000 Nvidia H800 GPUs, costing under 6 million. This is a fraction of the 6 million. This is a fraction of the 100 million OpenAI spent on GPT-4. DeepSeek’s ability to achieve high performance with minimal resources is a testament to its technical ingenuity.
Reduced Computational Time and Memory:
By deploying advanced strategies, DeepSeek significantly reduced the computational time and memory required to train and run its models. This not only lowers costs but addresses environmental concerns tied to AI’s massive energy consumption.
Side Project Origins:
DeepSeek began as a side project, showcasing how groundbreaking innovation can emerge from unconventional beginnings. This underdog story adds to its appeal and highlights the potential of grassroots innovation.
Hedge Fund Connection:
DeepSeek’s development is reportedly backed by hedge funds, providing it with the financial support needed to compete with well-established players. This connection has fueled its rapid growth and technological advancements.
NVIDIA Stock Price Impact:
DeepSeek’s announcement caused a dramatic drop in NVIDIA’s stock price, wiping out $600 billion in market value in a single day. This underscores the app’s disruptive potential and its ability to influence global markets.
The Secret Sauce: Efficiency and Innovation
DeepSeek’s success lies in its ability to achieve more with less. The company deployed cutting-edge technical strategies to reduce both the computational time required to train its R1 model and the memory needed to store it. For instance, the base model V3 required 2.788 million hours of training across 2,000 Nvidia H800 GPUs, costing under 6 million. In contrast, OpenAI’s GPT−4 reportedly cost over 100 million to train.
This efficiency isn’t just a win for DeepSeek’s bottom line; it also addresses growing concerns about the environmental impact of AI. Data centres powering AI models consume massive amounts of electricity and water, contributing to significant carbon footprints. By reducing computational costs, DeepSeek is paving the way for a more sustainable approach to AI development.
Reinforced Learning (RL): A Game-Changer
DeepSeek’s innovative use of reinforced learning (RL) sets it apart:
Problem-Solving Strategies:
RL enables AI to develop advanced problem-solving techniques, such as double-checking answers and reasoning through complex tasks.
Early Quirks and Fixes:
Early versions of the model, like R1-o, exhibited quirky behaviours like mixing languages. These were resolved by combining supervised learning with RL, resulting in a more structured and error-free approach.
Leadership in Advanced Reasoning:
DeepSeek’s focus on RL has positioned it as a leader in advanced reasoning tasks, particularly in mathematics, coding, and science.
The Road Ahead
DeepSeek’s rise is a testament to the power of innovation and efficiency in an industry often dominated by massive budgets and resources. With a development cost of just $6 million — compared to OpenAI’s 100 million and Gemini’s $200 million— DeepSeek has proven that groundbreaking advancements don’t always require astronomical investments.
However, its success raises important questions: Will its cost efficiency lead to increased, AI consumption, potentially offsetting its environmental benefits? How will established players like OpenAI and NVIDIA respond to this new challenger?
One thing is certain: DeepSeek has irrevocably changed the AI landscape. As the world watches this David-and-Goliath story unfold, one can’t help but wonder if this is just the beginning of a new era in artificial intelligence—one defined by accessibility, efficiency, and innovation.
For now, DeepSeek stands as a symbol of what’s possible when ingenuity meets determination, proving that even in the world of AI, the underdog can redefine the game.
Comments